Within the domain of machine learning, the term “ground truth” holds critical significance, what is ground truth in machine learning, serving as a foundation for show improvement, assessment, and approval? Ground truth alludes to the supreme, objective reality or adjusted reply in a given setting. It serves as a reference point against which the execution of machine learning calculations is measured. Let’s dive more profound into this concept to get its complexities and suggestions.
Presentation to Ground Truth
Ground truth speaks to the foremost dependable and precise information available around a specific marvel or space. Within the setting of machine learning, it gives a benchmark for evaluating the rightness and viability of prescient models. Whether it’s recognizing objects in pictures, translating sound recordings, or categorizing content reports, having to get to ground truth information is fundamental for preparing, testing, and refining machine learning calculations.
The Concept of Ground Truth in Machine Learning or What is Ground Truth in Machine Learning
In machine learning, ground truth information serves as the gold standard against which the execution of prescient models is assessed. It includes a range of properties, counting names, explanations, or target values, that characterize the right results for a given set of input information. What is Ground Truth in Machine Learning? This ground truth information is typically obtained through fastidious explanation by space specialists or other solid sources.
Challenges and Confinements
Whereas ground truth information is important for machine learning errands, getting it can be challenging and inclined to blunders. The method of manual explanation is labor-intensive and time-consuming, regularly leading to irregularities and mistakes. In addition, the subjective nature of certain tasks presents predispositions that will influence the quality of the ground truth.
Applications Different Areas
Ground truth plays an essential part in different spaces and applications of machine learning. In areas such as picture acknowledgment, common dialect preparation, healthcare, and independent vehicles, precise ground truth information is crucial for preparing vigorous and solid models that can generalize well to unseen information.
Strategies for Setting up Ground Truth
A few strategies are utilized to set up ground truth information, extending from manual explanation by human specialists to crowdsourcing and manufactured information era. What is Ground Truth in Machine Learning? Each approach has its qualities and confinements, and the choice of strategy depends on components such as the complexity of the information and the accessible assets.
Guaranteeing Quality and Unwavering quality
Keeping up the quality and unwavering quality of ground truth information is paramount to the victory of machine learning ventures. Approval strategies, such as cross-validation and inter-rater assertion, offer assistance evaluate the consistency and exactness of comments. Agreement among annotators and nonstop refinement of the ground truth is basic for improving its quality over time.
Ground Truth in Administered Learning
In administered learning assignments, ground truth information serves as the establishment for training machine learning models. By giving input-output sets comprising of highlights and corresponding names, ground truth empowers the demonstration to memorize the basic patterns and connections inside the information.
Assessing Demonstrate Execution
Once prepared, machine learning models are assessed based on their execution against the ground truth. Different measurements, such as exactness, accuracy, review, and F1-score, are utilized to assess the model’s capacity to create redress expectations. What is Ground Truth in Machine Learning? By comparing the model’s yields to the ground truth, analysts and specialists can gauge its adequacy and distinguish regions for change.
Moral Contemplations
Inclination and Decency
One of the essential moral concerns related to ground truth information is the nearness of predisposition, which can sustain imbalances and biased results in machine learning models. Inclination in ground truth may emerge from different sources, counting chronicled treacheries, social predispositions, and systemic imbalances. It’s basic to recognize and address predisposition in ground truth information through thorough approval, assorted representation, and fairness-aware calculations to guarantee evenhanded results for all people and communities.
Security Concerns
Another basic moral thought is the assurance of protection rights within the collection and utilization of ground truth information. Ground truth datasets frequently contain touchy data about people, such as individual identifiers, well-being records, and behavioral designs. What is Ground Truth in Machine Learning? Defending protection requires vigorous information anonymization methods, educated assent to conventions, and compliance with security controls, such as GDPR and HIPAA, to maintain individuals’ rights to security and information security.
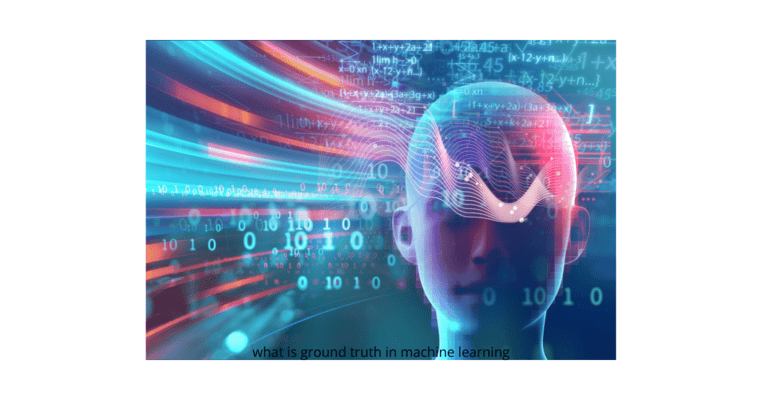
Straightforwardness and Responsibility
Straightforwardness and responsibility are crucial standards that support moral ground truth hones. Straightforwardness includes giving clear and available data around the beginning, reason, and utilization of ground truth data, enabling clients to create educated choices around its collection and utilization. Responsibility involves building up components for oversight, audibility, and change in case of abuse or manhandling of ground truth information, holding people and organizations responsible for their activities and choices.
Educated Assent and Cooperation
Individuals’ independence and office are basic when collecting ground truth information. Getting educated assent from members guarantees that they get the nature and suggestions of their association in information explanation exercises and have the opportunity to assent or withhold assent unreservedly. What is Ground Truth in Machine Learning? In addition, advancing inclusivity and differing qualities in ground truth comment endeavors cultivates important support and representation, improving the quality and validity of the information while minimizing the hazard of hurt or misuse.
Algorithmic Straightforwardness and Explainability
As machine learning models progressively depend on ground truth information for preparation and assessment, guaranteeing algorithmic straightforwardness and explainability becomes vital. Giving experiences into how models make choices and expectations make a difference in constructing belief and responsibility, empowering partners to get it, decipher, and challenge the results of AI frameworks. Methods such as demonstrate interpretability, reasonableness reviews, and affect appraisals encourage moral decision-making and moderate the dangers of unintended results or hurt.
Long-Term Societal Impacts
At last, moral contemplations expand past quick concerns to include the long-term societal impacts of ground truth information utilization. Expecting and tending to potential social, financial, and social suggestions of AI advances requires intriguing collaboration, partner engagement, and progressing exchange with influenced communities. By receiving a proactive and comprehensive approach to moral AI improvement, we can tackle the benefits of ground truth while minimizing its dangers and maximizing its positive effect on society.
Future Patterns and Improvements
Headways in Ground Truth Era
As innovation proceeds to advance, we are able anticipate to noteworthy progressions in ground truth era strategies. Advancements in counterfeit insights, machine learning, and computer vision will empower more productive and precise comments of information. What is Ground Truth in Machine Learning? Computerized annotation tools fueled by profound learning calculations will streamline the method of labeling expansive datasets, lessening the requirement for manual intercession and speeding up the advancement of machine learning models.
Integration with Profound Learning Procedures
The integration of ground truth information with profound learning methods will open up modern roads for investigation and improvement. Profound learning calculations, such as convolutional neural systems (CNNs) and repetitive neural systems (RNNs), exceed expectations of learning from huge sums of labeled information. By consolidating ground truth into the preparation, these calculations can accomplish higher levels of exactness and vigor, driving more dependable AI frameworks over different spaces.
Moral Systems and Controls
As the utilization of ground truth information gets to be more far-reaching, there will be an expanded center on moral considerations and regulatory systems. Concerns about predisposition, decency, and protection will drive endeavors to set up rules and benchmarks for the collection, utilization, and spread of ground truth information. Moral AI systems and directions will point to ensuring transparency, responsibility, and capable stewardship of information, shielding the rights and interface of people and communities.
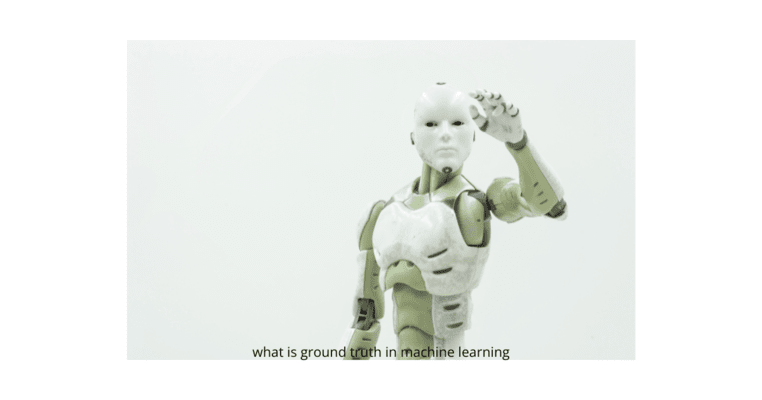
Real-time Ground Truth Comment
With the rise of real-time information streams and IoT gadgets, there will be a developing request for real-time ground truth explanation capabilities. Advances such as edge computing and combined learning will empower information to be clarified and labeled on the fly, permitting machine learning models to adjust and learn in real time. What is Ground Truth in Machine Learning? This will be especially important in applications such as independent vehicles, where opportune and exact ground truth is fundamental for secure and solid operation.
Expanded Reality and Virtual Reality
Increased reality (AR) and virtual reality (VR) innovations will play a progressively conspicuous part in the ground truth era. AR and VR situations give immersive and intelligent stages for explaining and labeling information, empowering clients to control and comment on 3D objects in virtual space. These innovations will encourage more instinctive and proficient comment workflows, upgrading the quality and lavishness of ground truth information for machine learning applications.
Collaborative Explanation Stages
Collaborative annotation stages will rise as central center points for the ground truth era and curation. These stages will bring together domain experts, data researchers, and machine learning analysts to collaboratively comment on and approve information. Highlights such as form control, explanation following, and consensus-building components will guarantee the quality and unwavering quality of ground truth datasets, cultivating collaboration and information sharing inside the AI community.
Conclusion
In conclusion, ground truth serves as the bedrock of machine learning, giving a reference point for show advancement, assessment, and approval. By understanding the concept of ground truth and its suggestions over different applications, analysts and professionals can saddle their control to construct more precise, dependable, and moral machine learning frameworks.
FAQs
What part does ground truth play in machine learning?
Ground truth serves as a benchmark for assessing the execution of machine learning models by giving reference information against which expectations are compared.
How can ground truth be built up for complex datasets?
Setting up ground truth for complex datasets frequently includes a combination of manual comments by space specialists, crowdsourcing, and engineered information era strategies.
What are the challenges related to ground truth explanation?
Challenges incorporate getting exact comments, tending to subjectivity and predisposition, and guaranteeing the consistency and unwavering quality of the ground truth information.
Is ground truth fundamental for all machine learning assignments?
Whereas ground truth is fundamental for administered learning errands, it may not be required for unsupervised or semi-supervised learning approaches, where the information may be unlabeled or in part labeled.
How can predispositions in ground truth information be tended to?
Inclinations in ground truth information can be moderated through the cautious choice of annotators, different representations within the explanation prepared, and thorough approval methods to identify and redress inclinations.